Risks of Synthetic Data in Education
1 min readSynthetic Data Is a Dangerous Teacher
Synthetic data, or artificially generated data, is increasingly being used in various industries for training machine learning models and...
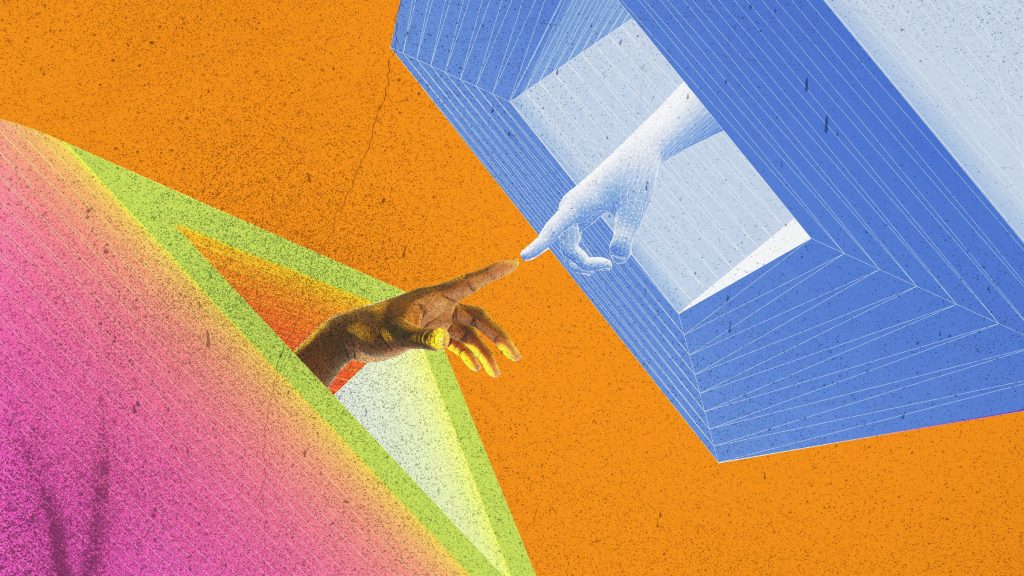
Synthetic Data Is a Dangerous Teacher
Synthetic data, or artificially generated data, is increasingly being used in various industries for training machine learning models and conducting research. While it may seem like a convenient solution to the problem of limited data availability, using synthetic data comes with its own set of risks and limitations.
One of the main concerns with synthetic data is that it may not accurately represent real-world data. Since synthetic data is generated based on assumptions and algorithms, it may not capture the complexities and nuances of the actual data it is meant to simulate. This can lead to unreliable results and a false sense of confidence in the model’s performance.
Furthermore, using synthetic data can also introduce biases and errors into the model. If the synthetic data is not representative of the true data distribution, the model may learn incorrect patterns and make inaccurate predictions. This can have serious consequences, especially in high-stakes applications such as healthcare or autonomous driving.
Therefore, while synthetic data can be a useful tool for supplementing limited datasets, it should not be relied upon as the sole source of training data. It is important to validate the performance of models trained on synthetic data using real-world data and to be aware of the potential pitfalls and limitations of using artificial data in machine learning.